Human Action Recognition in Videos Using Kinematic Features and Multiple Instance Learning
We propose a set of kinematic features that are derived from the optical flow, for human action recognition in videos. The set of kinematic features include divergence, vorticity, symmetric and anti-symmetric flow fields, second and third principal invariants of flow gradient and rate of strain tensor, and third principal invariant of rate of rotation tensor. Each kinematic feature, when computed from the optical flow of a sequence of images, gives rise to a spatio-temporal pattern. It is then assumed that the representative dynamics of the optical flow are captured by these spatio-temporal patterns in the form of dominant kinematic trends or kinematic modes. These kinematic modes are computed by performing Principal Component Analysis (PCA) on the spatio-temporal volumes of the kinematic features. For classification, we propose the use of multiple instance learning (MIL), in which each action video is represented by a bag of kinematic modes. Each video is then embedded into a kinematic mode-based feature space and the coordinates of the video in that space are used for classification using the nearest neighbor algorithm. The qualitative and quantitative results are reported on the benchmark data sets.
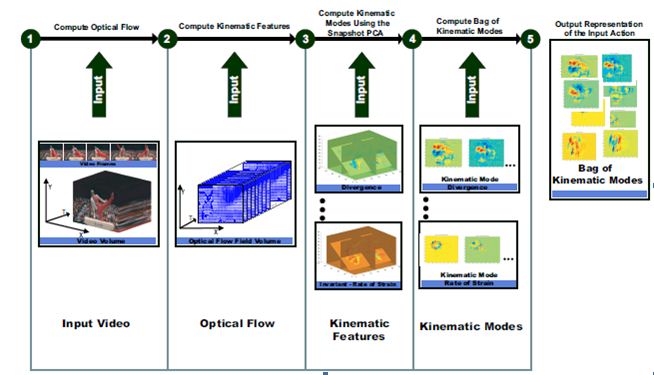